A two-step post-optimality approach for a multi-objective railway maintenance planning problem
Authors
Sanyapong Petchrompo, Sunny Modhara, Andy Kirwan, Ajith Kumar Parlikad, Naruemon Wattanapongsakorn
Journal
Computers & Industrial Engineering
Publication year
2024
Research summary
This paper addressed a railway asset management problem in which the decision maker (DM) is tasked with deriving a maintenance intervention plan to balance costs with multiple system performance objectives at the network level. We introduced a two-step approach to assist the DM in choosing a final solution in the post-optimality phase. The approach was devised based on the assumption that the DM cannot specify preferences before the optimization or directly choose a final solution from an extensive Pareto optimal set.
In the first step, Latent Profile Analysis (LPA) was employed to identify a small yet diverse set of representative solutions across the Pareto front. We demonstrated the efficacy of LPA in categorizing Pareto optimal solutions into distinct latent profiles. The numerical analysis also highlighted the ability of LPA to identify smaller, unique groups that do not align closely with larger clusters on the Pareto front. The application of LPA also facilitates the utilization of information criteria statistics to automatically determine the appropriate number of representative solutions within the initial pruned set.
In the second step, Data Envelopment Analysis (DEA) was employed to identify efficient solutions within the focus region supplied by the DM. Comparative analysis of CCR and BCC models indicated that the BCC model allowed more Decision Making Units (DMUs) to attain a relative efficiency score of 1, while the CCR model offered clearer distinctions in efficiency scores. Thus, in the case study, we recommended employing the CCR model due to its ability to provide precise solution ranking. The BCC model could also complement the CCR model if the primary aim is to eliminate inefficient solutions.
The proposed approach successfully offers the DM a promising solution from a large Pareto optimal set. It utilized diversity-based pruning to provide an overview of the Pareto front, allowing the DM to select a preferred region. Moreover, it identified the most efficient solution within the chosen area based on efficiency rankings. The two-step approach enhances data-driven decision-making while preserving human oversight in asset management. The final output of the model aided the DM in determining optimal objective values at the network level, facilitating informed maintenance plans at the operational level.
Graphical abstract
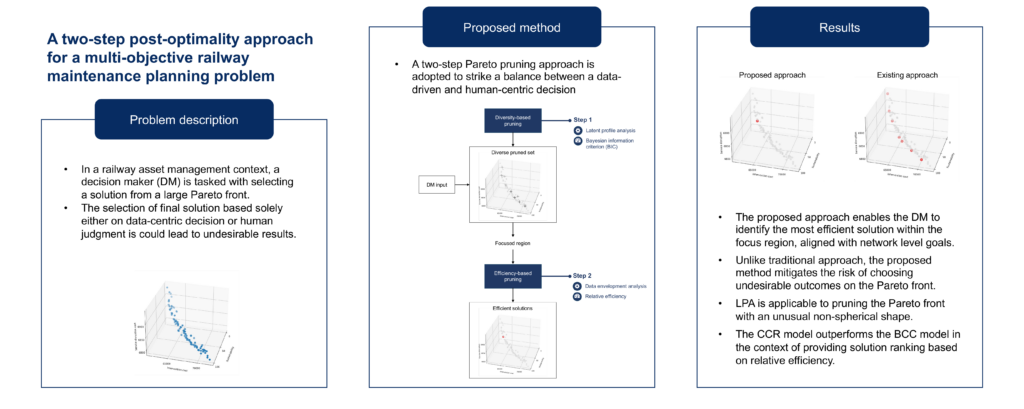